The (Quantum) Advantage of Conviction
This post is, tangentially, about investing in quantum computing. It’s for informational purposes, I’m just some stranger on the internet thinking out loud for your entertainment, this is not investing advice, etc. As I’ve mentioned before, I maintain a small long position in $IONQ.
A Lack of Conviction
As of November 18, 2021, IonQ has closed at $27.96 per share, up over ~40% from three days ago1 (and up nearly ~3x from their despac price of around $10). Since the deSPAC has anything fundamentally changed with the company? Third quarter earnings weren’t surprising or mindblowing, and the net operating loss was expected. In my mind, there is nothing fundamental2 standing between the current price and, say, a price of ~$5 per share3.
Although contemporary public equity markets appear to run mostly on narrative, there is always the question in my mind about what happens when the narrative juice runs out. So, naturally, I’ve been wondering about what happens after I buy my initial, speculative stake in some QC company. IonQ is the most recent, but the Rigetti SPAC has been announced and who knows which companies will follow suit soon.
The problem, simply stated, is that if Number Go Up is not sufficient reason (for me) to buy in further, then:
How would I know when it’s time to buy more IonQ?
You can substitute the name of your favorite QC company in there, I’m using $IONQ because it’s really the only publicly available option as of late 20214.
My answer would probably have a few criteria:
They demonstrate quantum advantage in some capacity/problem area.
They demonstrate a fault-tolerant logical qubit with effectively unlimited coherence and lifetime.
They announce one or more partnerships based on 1 & 2 above. Presumably these partnerships would be valued in the billions of USD.
Some sort of non-trivial technical steps toward 1 & 2 above.
I want to focus on item 1, because it doesn’t necessarily require fault-tolerance or real, logical qubits. Unfortunately, I’m a little worried that ‘Quantum Advantage’ will suffer from the same chimaerical elusiveness as ‘Quantum Supremacy’. In the latter case, although the authors of the original paper estimated a supercomputer would take 10,000 years to accomplish the same task their QPU did in a few seconds, recent pre-prints have shown that the rumors of classical computing’s demise have been greatly exaggerated.
The original, somewhat vague, question has been sharpened and transformed into:
What is ‘Quantum Advantage’ and how do I recognize it?
This is still a tricky one, since the answer likely depends on the space of problems we are trying to solve with our QPU. Providence has smiled upon us, since I have recently stumbled across a pre-print from 2020 where this question is posed for quantum chemistry.
It’s a dense paper, riddled with quantum chemistry jargon, but you don’t have to be a computational quantum chemist to get the main points. After reading through it, I ended up with a few criteria for recognizing steps toward quantum advantage in computational chemistry.
Bigger Basis Sets
Pretty much all articles in which some molecule is simulated on a quantum computer are using the smallest, simplest possible basis sets. This essentially precludes these sims from reaching chemical accuracy, as the first figure from the paper shows. Larger basis sets will require more qubits and much more run time, but are also sine qua non for quantum advantage. I think an interesting thing to do for new chemistry results on QPUs is just to look up the most recent paper simulating the same molecule classically, and comparing the details of the basis sets and other computational benchmarks.
A nice feature of the quantum chemistry basis sets is that there are so many of them. At first, this is annoying because the nomenclature looks like alphabet soup (STO-nG vs 6-31G vs cc-pVQZ), but there appears to be method to the madness. The many flavors of basis set encode not only # of electrons and orbitals in the models, but also appear to account for important physical effects required to model these molecules accurately. I suspect you can track the quality of quantum molecular simulation almost solely by the evolution of the basis sets in use.
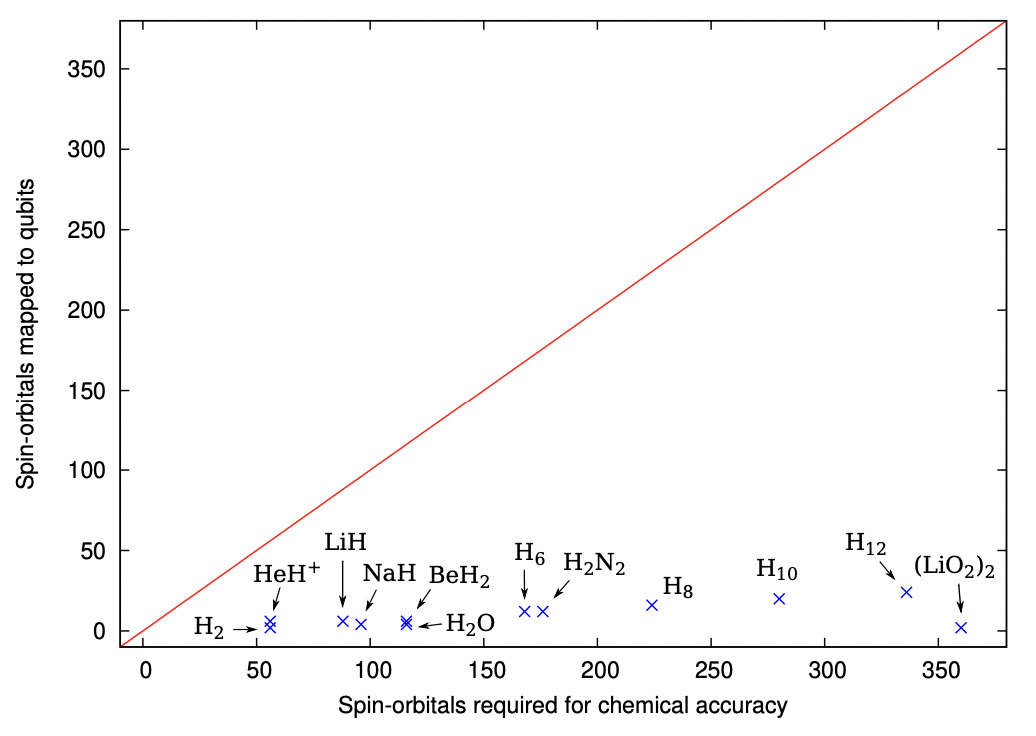
Relevant Problem Selection
The molecules modeled on QPUs so far can be solved pretty quickly using classical techniques. I would want to see a steady march to more difficult molecules up to the chromium dimer, which divides (arbitrarily?) the classically tractable from the quantum computationally interesting. Incidentally, the authors estimate that Cr2 simulated on a quantum computer would take on the order of a few million qubits and between 5-40 days at currently achievable error rates. The magnitude of the task ahead is substantial.
You can see at the far right end of the figure below are ‘homogeneous catalysts’ and FeMoCo. The former are identified as molecules of great relevance to industrial applications, and FeMoCo of course, is almost the holy grail of quantum molecular simulations. The authors consider homogeneous catalysts to be the most promising near-term category for quantum computers to tackle. The have this to say:
An attractive subclass of homogeneous catalysts on which early quantum advantage studies can be focused are biomimetics. These normally di- and tri-metal complexes borrow chemical insight from natural, metal containing enzymes and are designed for tackling industrially important chemical transformations such as C-H bond activation or the N2 bond cleavage. Highly efficient C-H bond activation, for example, is at the heart of the idea of “methanol economy”, which seeks to replace petroleum and coal by cleaner sources of energy and synthetic materials.
Right now, the motivation for quantum simulation often boils down to ‘FeMoCo, someday’. I would expect, as the technology improves and matures, more papers will explicitly target some easier class of relevant molecules, perhaps the homogeneous catalysts, perhaps something else, as their motivation.
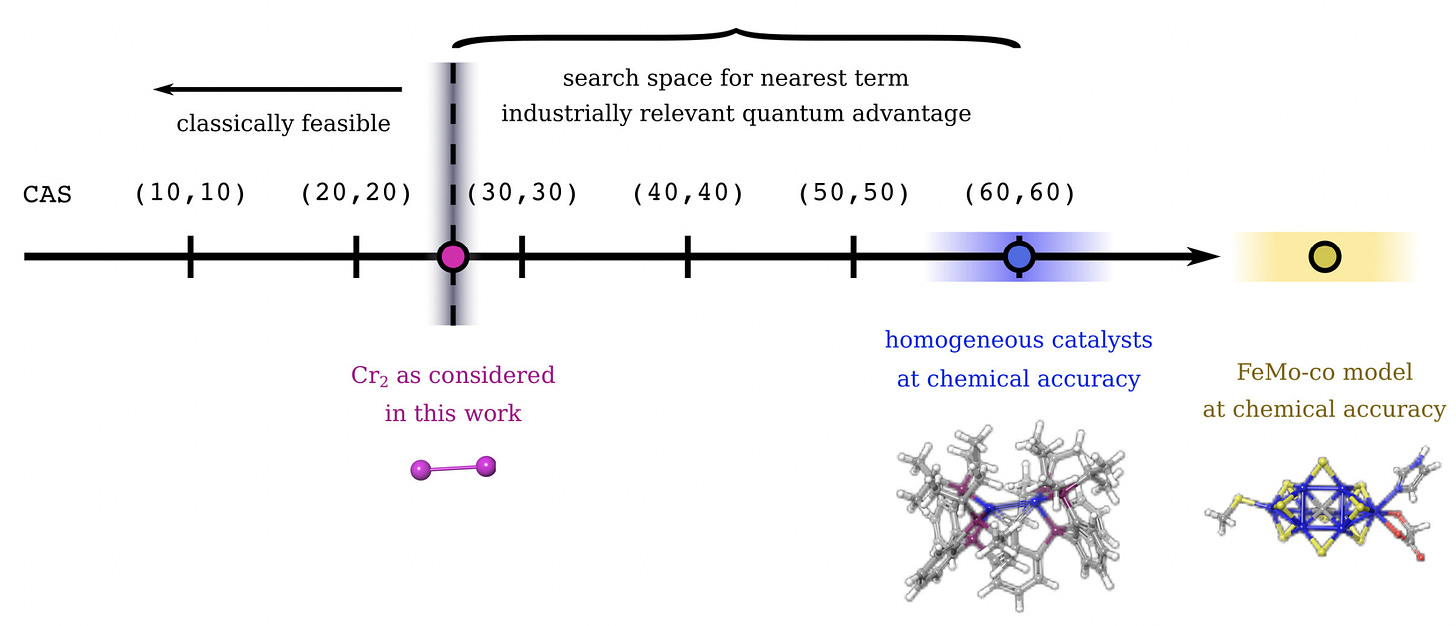
The two points above are chemistry specific, but there are also two more general criteria that should be applicable across many fields.
Hardware Overhead Minimized
Large prefactors on polynomial run-times may rob us of exponential speedups. This is due to the specter of substantial hardware overhead for early fault-tolerant schemes. The authors address this directly in the conclusion:
Even though an exponential speedup of quantum chemical calculations is theoretically expected on quantum hardware, a significant obstacle to consider is the enormous prefactor to the polynomial runtime of quantum computational algorithms. This prefactor is partially due to the desired chemical accuracy requiring a long circuit decomposition in the gate-based model, but primarily it is due to the enormous overhead that fault tolerant error correcting codes require.
In general, this overhead should be reduced by higher quality qubits and higher quality gates, which will reduce the amount of hardware per logical qubit. There may also be improvements on error correcting codes that offer substantial reductions in the physical qubit to logical qubit ratio. To that end, it’s important to keep an eye on the field, and maybe keep a tame quantum error correction theorist nearby to parse recent results.
Classical ←→ Quantum bottlenecks identified
Part of simulating molecules includes doing a lot of two-electron integrals. The number of such integrals scales as “the fourth power of the molecular size”. The authors assert that computing these integrals for 1,000 orbitals creates about 1 TB of data. Bottlenecks moving this data around, or recalculating it could be costly, and will certainly have to be considered as algorithms and use-cases are evaluated.
This is more of an architecture problem, I think. As far as I know quantum computing architectures are also a vigorous area of research, but one that I’m less plugged into than I’d like. Given the huge differences in between various types of qubit, I wouldn’t expect all architectures to be equally applicable across QC approaches, at least not in the near term. I’m not sure how good I’d be at identifying real architecture improvements, which just feeds into my distress that all of life is fractally uncertain and you can never know anything.
Outlook
I’m still somewhat uncertain in my ability to recognize an imminent quantum advantage in chemical simulation, but I do feel more confident after this exercise. Before reading the paper and writing this post, I generally felt that I had to take results mostly on faith, since I knew nothing about the field. Afterward, though, I feel better able to critically engage with the literature and better evaluate what actual progress is being made toward quantum advantage. I’m looking forward to reading future quantum chemical simulation papers with a more critical eye.
Down about 9.8% from Nov 17, 2021 close.
Yeah yeah, you can argue fundamentals are meaningless, the real game is narrative, etc. Call me old fashioned, but I expect reality to eventually reassert itself.
Realistically, the floor of the share price could reasonably be said to be the total value of all of their tangible assets divided by number of shares. I don’t know what that figure is, exactly (because researching that is boring), but I think we can all agree that number is « $1 billion.